Abstract:
The proliferation of the Internet of Things (IoT), artificial intelligence (AI), the adoption of 5G, and progress towards 6G technology have led to the accumulation of massive amounts of real-world data; however, a significant portion of the data generated by smart cities and smart buildings remains unused. A notable problem is the shift of statistical properties in real-world streaming over time caused by unexpected factors, referred to as concept drift, which results in less efficient predictive models. To address this problem, the latest research leverages the cloud–edge continuum paradigm for the deployment of AI and general smart city applications while utilising the available resources optimally. In this article, we propose a computing architecture for different smart city applications in edge micro data centre (EMDC) settings over a hybrid cloud–edge continuum to support the deployment of AI workloads. We implement a feedback-driven automated concept drift detection and adaptation methodology, combining base learner long short-term memory (LSTM) with Page– Hinkley test (PHT), daptive windowing (ADWIN) and the Kolmogorov–Smirnov windowing (KSWIN). Real-world data streams are utilised to forecast from various environmental sensors installed at the University of Oulu Smart Campus. The feedback-based concept drift detection and adaption process
is first evaluated using synthetic datasets with known concept drift points and then employed in the real-world data. Subsequently, the implementation is evaluated using the state-of-the-art MAE, RMSE, and MAPE methods. The results showed a reduction in MAPE from 8.5% to 3.88% when concept drift detection was applied. Additionally, the challenges faced and the effectiveness of the suggested solutions are explored.
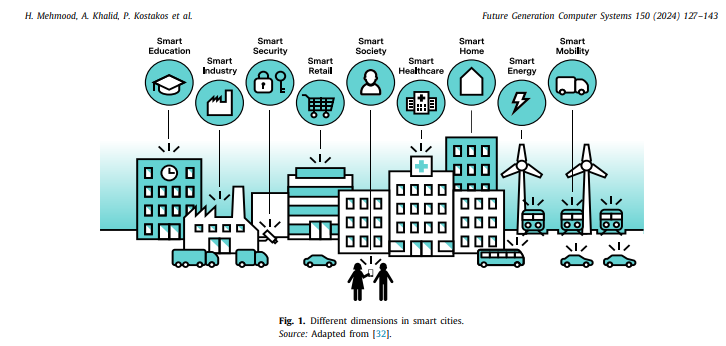